At the American Astronomical Society meeting in Washington DC last month (January was loooooong subjectively this year), I sat in a fascinating talk given by Karteyk Iyer. He and his collaborators developed a clustering map of the astrophysics literature. Quite literally the current landscape of the field.
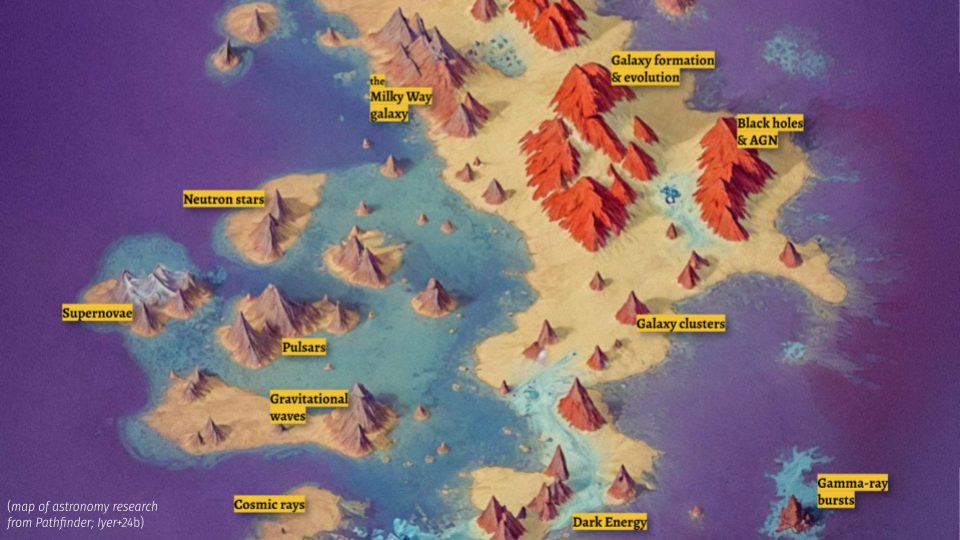
This maps out where lots (enough?) has been said already and where more could be done.
To show this in a little more scientific heat map:
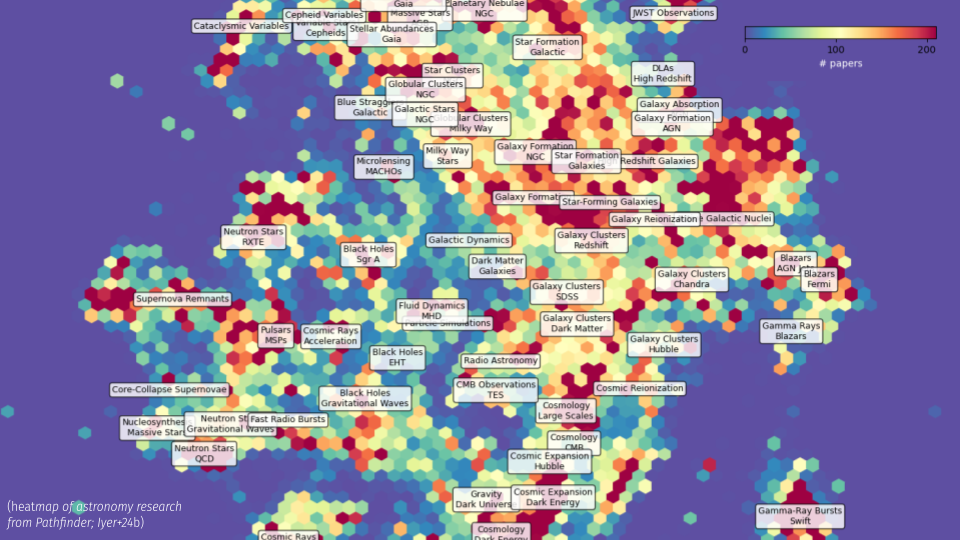
What made me perk up about this was the option to ask a question or put in a phrase and get a list of papers that talk about that subject. This is partly LLM based and therefore can interpret plain language.
This hopefully circumvents the problem that certain issues had different nomenclature. For example “dark matter” (Vera Rubin’s and earlier Zwicky’s phrase) was talked about as “non-stellar mass-to-light ratio” by the radio astronomers. In effect the same thing. A sufficiently trained LLM can perhaps circumvent that.
You can try this here:
https://huggingface.co/spaces/kiyer/pathfinder
On the one hand this makes me very excited. Putting in a phrase as part of my paper followed by a bunch of citations always elicited a worry that I was overlooking someone’s work unfairly. Simply because my memory of who did what is fallible at best and completely out to lunch at worst. I’m not alone in this, part of a referee’s job is to suggest more references that are relevant to the subject at hand. Better but still very fallible. I have tried some other tools (use both google scholar and ADS for example, connectedpapers was another).
And it has been shown that for example women are cited less in science (Nature: https://www.nature.com/articles/s41586-022-04966-w). I can imagine nothing more nefarious than poor human memory is to blame. I can also imagine other reasons.
So I was excited at the possibility of at least improving this issue of fair citations. One can put in the phrase that preceded the citations and see if more references pop up. Maybe to jog your memory.
This would be virtuous (give proper credit) but also make it easy to do (easy virtue….wait…).
The list often includes false positives I’ve found while playing around with it. It’s ranking of most relevant works certainly did not align with my personal one (that is also very subjective, which paper convinced you has something to do with which one you encountered first).
There is also no way to include feedback. And with many AI/ML applications, this is all dependent on the ingested training sample. A lot of science has been done before 1995 for example but may not be part of the ADS data-base. Has every journal been included? Some poorer academics only publish their results/catalogs on astro-ph because that is all they can afford (preprints not included right now), what was in a textbook etc etc.
But this shiny new AI tool may help me improve my citation practice. It might help yours as well.